SCEDC Tutorial#
Noisepy is a python software package to process ambient seismic noise cross correlations. This tutorial aims to introduce the use of noisepy for a toy problem on the SCEDC data. It can be ran locally or on the cloud.
The data is stored on AWS S3 as the SCEDC Data Set: https://scedc.caltech.edu/data/getstarted-pds.html
First, we install the noisepy-seis package
# Uncomment and run this line if the environment doesn't have noisepy already installed:
# ! pip install noisepy-seis
Warning: NoisePy uses obspy
as a core Python module to manipulate seismic data. If you use Google Colab, restart the runtime now for proper installation of obspy
on Colab.
Import necessary modules#
Then we import the basic modules
%load_ext autoreload
%autoreload 2
from noisepy.seis import cross_correlate, stack_cross_correlations, __version__ # noisepy core functions
from noisepy.seis.io.asdfstore import ASDFCCStore, ASDFStackStore # Object to store ASDF data within noisepy
from noisepy.seis.io.s3store import SCEDCS3DataStore # Object to query SCEDC data from on S3
from noisepy.seis.io.channel_filter_store import channel_filter
from noisepy.seis.io.datatypes import CCMethod, ConfigParameters, FreqNorm, RmResp, StackMethod, TimeNorm # Main configuration object
from noisepy.seis.io.channelcatalog import XMLStationChannelCatalog # Required stationXML handling object
import os
import shutil
from datetime import datetime, timezone
from datetimerange import DateTimeRange
from noisepy.seis.io.plotting_modules import plot_all_moveout
print(f"Using NoisePy version {__version__}")
path = "./scedc_data"
os.makedirs(path, exist_ok=True)
cc_data_path = os.path.join(path, "CCF")
stack_data_path = os.path.join(path, "STACK")
S3_STORAGE_OPTIONS = {"s3": {"anon": True}}
/opt/hostedtoolcache/Python/3.10.16/x64/lib/python3.10/site-packages/noisepy/seis/io/utils.py:13: TqdmExperimentalWarning: Using `tqdm.autonotebook.tqdm` in notebook mode. Use `tqdm.tqdm` instead to force console mode (e.g. in jupyter console)
from tqdm.autonotebook import tqdm
Using NoisePy version 0.1.dev1
We will work with a single day worth of data on SCEDC. The continuous data is organized with a single day and channel per miniseed (https://scedc.caltech.edu/data/cloud.html). For this example, you can choose any year since 2002. We will just cross correlate a single day.
# SCEDC S3 bucket common URL characters for that day.
S3_DATA = "s3://scedc-pds/continuous_waveforms/"
# timeframe for analysis
start = datetime(2002, 1, 1, tzinfo=timezone.utc)
end = datetime(2002, 1, 2, tzinfo=timezone.utc)
timerange = DateTimeRange(start, end)
print(timerange)
2002-01-01T00:00:00+0000 - 2002-01-02T00:00:00+0000
The station information, including the instrumental response, is stored as stationXML in the following bucket
S3_STATION_XML = "s3://scedc-pds/FDSNstationXML/CI/" # S3 storage of stationXML
Ambient Noise Project Configuration#
We prepare the configuration of the workflow by declaring and storing parameters into the ConfigParameters()
object and/or editing the config.yml
file.
# Initialize ambient noise workflow configuration
config = ConfigParameters() # default config parameters which can be customized
Customize the job parameters below:
config.start_date = start
config.end_date = end
config.acorr_only = False # only perform auto-correlation or not
config.xcorr_only = True # only perform cross-correlation or not
config.inc_hours = 24 # INC_HOURS is used in hours (integer) as the
#chunk of time that the paralelliztion will work.
# data will be loaded in memory, so reduce memory with smaller
# inc_hours if there are over 400+ stations.
# At regional scale for SCEDC, we can afford 20Hz data and inc_hour
# being a day of data.
# pre-processing parameters
config.sampling_rate = 20 # (int) Sampling rate in Hz of desired processing (it can be different than the data sampling rate)
config.single_freq = False
config.cc_len = 3600 # (float) basic unit of data length for fft (sec)
config.step = 1800.0 # (float) overlapping between each cc_len (sec)
config.ncomp = 3 # 1 or 3 component data (needed to decide whether do rotation)
config.stationxml = False # station.XML file used to remove instrument response for SAC/miniseed data
# If True, the stationXML file is assumed to be provided.
config.rm_resp = RmResp.INV # select 'no' to not remove response and use 'inv' if you use the stationXML,'spectrum',
############## NOISE PRE-PROCESSING ##################
config.freqmin,config.freqmax = 0.05,2.0 # broad band filtering of the data before cross correlation
config.max_over_std = 10 # threshold to remove window of bad signals: set it to 10*9 if prefer not to remove them
################### SPECTRAL NORMALIZATION ############
config.freq_norm = FreqNorm.RMA # choose between "rma" for a soft whitening or "no" for no whitening. Pure whitening is not implemented correctly at this point.
config.smoothspect_N = 10 # moving window length to smooth spectrum amplitude (points)
# here, choose smoothspect_N for the case of a strict whitening (e.g., phase_only)
#################### TEMPORAL NORMALIZATION ##########
config.time_norm = TimeNorm.ONE_BIT # 'no' for no normalization, or 'rma', 'one_bit' for normalization in time domain,
config.smooth_N = 10 # moving window length for time domain normalization if selected (points)
############ cross correlation ##############
config.cc_method = CCMethod.XCORR # 'xcorr' for pure cross correlation OR 'deconv' for deconvolution;
# FOR "COHERENCY" PLEASE set freq_norm to "rma", time_norm to "no" and cc_method to "xcorr"
# OUTPUTS:
config.substack = True # True = smaller stacks within the time chunk. False: it will stack over inc_hours
config.substack_windows = 1 # how long to stack over (for monitoring purpose)
# if substack=True, substack_windows=2, then you pre-stack every 2 correlation windows.
# for instance: substack=True, substack_windows=1 means that you keep ALL of the correlations
# if substack=False, the cross correlation will be stacked over the inc_hour window
### For monitoring applications ####
## we recommend substacking ever 2-4 cross correlations and storing the substacks
# e.g.
# config.substack = True
# config.substack_windows = 4
config.maxlag = 200 # lags of cross-correlation to save (sec)
# For this tutorial make sure the previous run is empty
#os.system(f"rm -rf {cc_data_path}")
if os.path.exists(cc_data_path):
shutil.rmtree(cc_data_path)
Step 1: Cross-correlation#
In this instance, we read directly the data from the scedc bucket into the cross correlation code. We are not attempting to recreate a data store. Therefore we go straight to step 1 of the cross correlations.
We first declare the data and cross correlation stores
config.networks = ["CI"]
config.stations = ["RPV", "SVD", "BBR"]
config.channels = ["BH?"]
catalog = XMLStationChannelCatalog(S3_STATION_XML, storage_options=S3_STORAGE_OPTIONS) # Station catalog
raw_store = SCEDCS3DataStore(S3_DATA, catalog,
channel_filter(config.networks, config.stations, config.channels),
timerange, storage_options=S3_STORAGE_OPTIONS) # Store for reading raw data from S3 bucket
cc_store = ASDFCCStore(cc_data_path) # Store for writing CC data
get the time range of the data in the data store inventory
span = raw_store.get_timespans()
print(span)
[2002-01-01T00:00:00+0000 - 2002-01-02T00:00:00+0000]
Get the channels available during a given time spane
channel_list=raw_store.get_channels(span[0])
print(channel_list)
2025-01-14 01:10:20,851 139664327617408 INFO utils.log_raw(): TIMING: 0.383 secs for Listing 877 files from s3://scedc-pds/continuous_waveforms/2002/2002_001/
2025-01-14 01:10:20,870 139664327617408 INFO utils.log_raw(): TIMING: 0.018 secs for Init: 1 timespans and 9 channels
2025-01-14 01:10:21,014 139662825883328 INFO channelcatalog._get_inventory_from_file(): Reading StationXML file s3://scedc-pds/FDSNstationXML/CI/CI_SVD.xml
2025-01-14 01:10:21,173 139662718928576 INFO channelcatalog._get_inventory_from_file(): Reading StationXML file s3://scedc-pds/FDSNstationXML/CI/CI_RPV.xml
2025-01-14 01:10:21,175 139662737802944 INFO channelcatalog._get_inventory_from_file(): Reading StationXML file s3://scedc-pds/FDSNstationXML/CI/CI_BBR.xml
2025-01-14 01:10:24,212 139664327617408 INFO s3store.get_channels(): Getting 9 channels for 2002-01-01T00:00:00+0000 - 2002-01-02T00:00:00+0000
[CI.BBR.BHE, CI.BBR.BHN, CI.BBR.BHZ, CI.RPV.BHE, CI.RPV.BHN, CI.RPV.BHZ, CI.SVD.BHE, CI.SVD.BHN, CI.SVD.BHZ]
Perform the cross correlation#
The data will be pulled from SCEDC, cross correlated, and stored locally if this notebook is ran locally.
If you are re-calculating, we recommend to clear the old cc_store
.
cross_correlate(raw_store, config, cc_store)
2025-01-14 01:10:24,261 139664327617408 INFO correlate.cross_correlate(): Starting Cross-Correlation with 4 cores
2025-01-14 01:10:24,263 139664327617408 INFO s3store.get_channels(): Getting 9 channels for 2002-01-01T00:00:00+0000 - 2002-01-02T00:00:00+0000
2025-01-14 01:10:24,268 139664327617408 INFO utils.log_raw(): TIMING CC Main: 0.006 secs for get 9 channels
2025-01-14 01:10:24,269 139664327617408 INFO correlate.cc_timespan(): Checking for stations already done: 6 pairs
2025-01-14 01:10:24,270 139664327617408 INFO utils.log_raw(): TIMING CC Main: 0.002 secs for check for 3 stations already done (warm up cache)
2025-01-14 01:10:24,272 139664327617408 INFO utils.log_raw(): TIMING CC Main: 0.001 secs for check for stations already done
2025-01-14 01:10:24,272 139664327617408 INFO correlate.cc_timespan(): Still need to process: 3/3 stations, 9/9 channels, 6/6 pairs for 2002-01-01T00:00:00+0000 - 2002-01-02T00:00:00+0000
2025-01-14 01:10:25,158 139664327617408 INFO correlate._filter_channel_data(): Filtered to 9/9 channels with sampling rate >= 20.0
2025-01-14 01:10:25,159 139664327617408 INFO utils.log_raw(): TIMING CC Main: 0.888 secs for Read channel data: 9 channels
2025-01-14 01:10:25,741 139662297401024 INFO noise_module.preprocess_raw(): removing response for CI.RPV..BHN | 2002-01-01T00:00:00.010758Z - 2002-01-01T23:59:59.960758Z | 20.0 Hz, 1728000 samples using inv
2025-01-14 01:10:25,750 139662316275392 INFO noise_module.preprocess_raw(): removing response for CI.BBR..BHN | 2002-01-01T00:00:00.025573Z - 2002-01-01T23:59:59.975573Z | 20.0 Hz, 1728000 samples using inv
2025-01-14 01:10:25,781 139662114948800 INFO noise_module.preprocess_raw(): removing response for CI.BBR..BHZ | 2002-01-01T00:00:00.025573Z - 2002-01-01T23:59:59.975573Z | 20.0 Hz, 1728000 samples using inv
2025-01-14 01:10:25,796 139662335149760 INFO noise_module.preprocess_raw(): removing response for CI.SVD..BHZ | 2002-01-01T00:00:00.048083Z - 2002-01-01T23:59:59.998083Z | 20.0 Hz, 1728000 samples using inv
2025-01-14 01:10:25,797 139662532282048 INFO noise_module.preprocess_raw(): removing response for CI.SVD..BHE | 2002-01-01T00:00:00.048083Z - 2002-01-01T23:59:59.998083Z | 20.0 Hz, 1728000 samples using inv
2025-01-14 01:10:25,814 139662494533312 INFO noise_module.preprocess_raw(): removing response for CI.SVD..BHN | 2002-01-01T00:00:00.048083Z - 2002-01-01T23:59:59.998083Z | 20.0 Hz, 1728000 samples using inv
2025-01-14 01:10:25,832 139662133823168 INFO noise_module.preprocess_raw(): removing response for CI.RPV..BHE | 2002-01-01T00:00:00.010758Z - 2002-01-01T23:59:59.960758Z | 20.0 Hz, 1728000 samples using inv
2025-01-14 01:10:25,844 139662513407680 INFO noise_module.preprocess_raw(): removing response for CI.BBR..BHE | 2002-01-01T00:00:00.025573Z - 2002-01-01T23:59:59.975573Z | 20.0 Hz, 1728000 samples using inv
2025-01-14 01:10:34,834 139662532282048 INFO noise_module.preprocess_raw(): removing response for CI.RPV..BHZ | 2002-01-01T00:00:00.010758Z - 2002-01-01T23:59:59.960758Z | 20.0 Hz, 1728000 samples using inv
2025-01-14 01:11:03,233 139664327617408 INFO utils.log_raw(): TIMING CC Main: 38.073 secs for Preprocess: 9 channels
2025-01-14 01:11:03,234 139664327617408 INFO correlate.check_memory(): Require 0.11gb memory for cross correlations
2025-01-14 01:11:04,210 139664327617408 INFO utils.log_raw(): TIMING CC Main: 0.976 secs for Compute FFTs: 9 channels
2025-01-14 01:11:04,212 139664327617408 INFO correlate.cc_timespan(): Starting CC with 6 station pairs
2025-01-14 01:11:05,820 139664327617408 INFO utils.log_raw(): TIMING CC Main: 1.608 secs for Correlate and write to store
2025-01-14 01:11:05,975 139664327617408 INFO utils.log_raw(): TIMING CC Main: 41.713 secs for Process the chunk of 2002-01-01T00:00:00+0000 - 2002-01-02T00:00:00+0000
2025-01-14 01:11:05,982 139664327617408 INFO utils.log_raw(): TIMING CC Main: 41.721 secs for Step 1 in total with 4 cores
The cross correlations are saved as a single file for each channel pair and each increment of inc_hours. We now will stack all the cross correlations over all time chunk and look at all station pairs results.
Step 2: Stack the cross correlation#
We now create the stack stores. Because this tutorial runs locally, we will use an ASDF stack store to output the data. ASDF is a data container in HDF5 that is used in full waveform modeling and inversion. H5 behaves well locally.
Each station pair will have 1 H5 file with all components of the cross correlations. While this produces many H5 files, it has come down to the noisepy team’s favorite option:
the thread-safe installation of HDF5 is not trivial
the choice of grouping station pairs within a single file is not flexible to a broad audience of users.
# open a new cc store in read-only mode since we will be doing parallel access for stacking
cc_store = ASDFCCStore(cc_data_path, mode="r")
stack_store = ASDFStackStore(stack_data_path)
Configure the stacking#
There are various methods for optimal stacking. We refern to Yang et al (2022) for a discussion and comparison of the methods:
Yang X, Bryan J, Okubo K, Jiang C, Clements T, Denolle MA. Optimal stacking of noise cross-correlation functions. Geophysical Journal International. 2023 Mar;232(3):1600-18. https://doi.org/10.1093/gji/ggac410
Users have the choice to implement linear, phase weighted stacks pws (Schimmel et al, 1997), robust stacking (Yang et al, 2022), acf autocovariance filter (Nakata et al, 2019), nroot stacking. Users may choose the stacking method of their choice by entering the strings in config.stack_method
.
If chosen all, the current code only ouputs linear, pws, robust since nroot is less common and acf is computationally expensive.
config.stack_method = StackMethod.LINEAR
method_list = [method for method in dir(StackMethod) if not method.startswith("__")]
print(method_list)
['ALL', 'AUTO_COVARIANCE', 'LINEAR', 'NROOT', 'PWS', 'ROBUST', 'SELECTIVE']
cc_store.get_station_pairs()
[(CI.RPV, CI.RPV),
(CI.BBR, CI.BBR),
(CI.SVD, CI.RPV),
(CI.BBR, CI.RPV),
(CI.SVD, CI.BBR),
(CI.SVD, CI.SVD)]
stack_cross_correlations(cc_store, stack_store, config)
2025-01-14 01:11:06,111 139664327617408 INFO stack.initializer(): Station pairs: 6
2025-01-14 01:11:09,681 140502672092032 INFO stack.stack_store_pair(): Stacking CI.BBR_CI.BBR/2002-01-01T00:00:00+0000 - 2002-01-02T00:00:00+0000
2025-01-14 01:11:09,703 140168518065024 INFO stack.stack_store_pair(): Stacking CI.BBR_CI.RPV/2002-01-01T00:00:00+0000 - 2002-01-02T00:00:00+0000
2025-01-14 01:11:09,711 140502672092032 INFO utils.log_raw(): TIMING: 0.026 secs for loading CCF data
2025-01-14 01:11:09,715 140147221670784 INFO stack.stack_store_pair(): Stacking CI.RPV_CI.RPV/2002-01-01T00:00:00+0000 - 2002-01-02T00:00:00+0000
2025-01-14 01:11:09,720 140502672092032 INFO utils.log_raw(): TIMING: 0.009 secs for stack/rotate all station pairs (CI.BBR, CI.BBR)
2025-01-14 01:11:09,738 140502672092032 INFO utils.log_raw(): TIMING: 0.018 secs for writing stack pair (CI.BBR, CI.BBR)
2025-01-14 01:11:09,741 140502672092032 INFO stack.stack_store_pair(): Stacking CI.SVD_CI.RPV/2002-01-01T00:00:00+0000 - 2002-01-02T00:00:00+0000
2025-01-14 01:11:09,742 140168518065024 INFO utils.log_raw(): TIMING: 0.036 secs for loading CCF data
2025-01-14 01:11:09,745 140147221670784 INFO utils.log_raw(): TIMING: 0.027 secs for loading CCF data
2025-01-14 01:11:09,755 140147221670784 INFO utils.log_raw(): TIMING: 0.010 secs for stack/rotate all station pairs (CI.RPV, CI.RPV)
2025-01-14 01:11:09,755 140168518065024 INFO utils.log_raw(): TIMING: 0.013 secs for stack/rotate all station pairs (CI.BBR, CI.RPV)
2025-01-14 01:11:09,774 140279805860736 INFO stack.stack_store_pair(): Stacking CI.SVD_CI.BBR/2002-01-01T00:00:00+0000 - 2002-01-02T00:00:00+0000
2025-01-14 01:11:09,774 140147221670784 INFO utils.log_raw(): TIMING: 0.019 secs for writing stack pair (CI.RPV, CI.RPV)
2025-01-14 01:11:09,774 140147221670784 INFO stack.stack_store_pair(): Stacking CI.SVD_CI.SVD/2002-01-01T00:00:00+0000 - 2002-01-02T00:00:00+0000
2025-01-14 01:11:09,780 140502672092032 INFO utils.log_raw(): TIMING: 0.037 secs for loading CCF data
2025-01-14 01:11:09,794 140502672092032 INFO utils.log_raw(): TIMING: 0.014 secs for stack/rotate all station pairs (CI.SVD, CI.RPV)
2025-01-14 01:11:09,805 140147221670784 INFO utils.log_raw(): TIMING: 0.029 secs for loading CCF data
2025-01-14 01:11:09,809 140168518065024 INFO utils.log_raw(): TIMING: 0.053 secs for writing stack pair (CI.BBR, CI.RPV)
2025-01-14 01:11:09,814 140147221670784 INFO utils.log_raw(): TIMING: 0.009 secs for stack/rotate all station pairs (CI.SVD, CI.SVD)
2025-01-14 01:11:09,823 140279805860736 INFO utils.log_raw(): TIMING: 0.042 secs for loading CCF data
2025-01-14 01:11:09,825 140147221670784 INFO utils.log_raw(): TIMING: 0.011 secs for writing stack pair (CI.SVD, CI.SVD)
2025-01-14 01:11:09,835 140279805860736 INFO utils.log_raw(): TIMING: 0.012 secs for stack/rotate all station pairs (CI.SVD, CI.BBR)
2025-01-14 01:11:09,848 140502672092032 INFO utils.log_raw(): TIMING: 0.054 secs for writing stack pair (CI.SVD, CI.RPV)
2025-01-14 01:11:09,871 140279805860736 INFO utils.log_raw(): TIMING: 0.036 secs for writing stack pair (CI.SVD, CI.BBR)
2025-01-14 01:11:10,416 139664327617408 INFO utils.log_raw(): TIMING: 4.307 secs for step 2 in total
QC_1 of the cross correlations for Imaging#
We now explore the quality of the cross correlations. We plot the moveout of the cross correlations, filtered in some frequency band.
cc_store.get_station_pairs()
[(CI.RPV, CI.RPV),
(CI.BBR, CI.BBR),
(CI.SVD, CI.RPV),
(CI.BBR, CI.RPV),
(CI.SVD, CI.BBR),
(CI.SVD, CI.SVD)]
pairs = stack_store.get_station_pairs()
print(f"Found {len(pairs)} station pairs")
sta_stacks = stack_store.read_bulk(timerange, pairs) # no timestamp used in ASDFStackStore
Found 6 station pairs
2025-01-14 01:11:10,584 139664327617408 INFO utils.log_raw(): TIMING: 0.115 secs for loading 6 stacks
plot_all_moveout(sta_stacks, 'Allstack_linear', 0.1, 0.2, 'ZZ', 1)
2025-01-14 01:11:10,609 139664327617408 INFO plotting_modules.plot_all_moveout(): Plottting: Allstack_linear, 6 station pairs
200 8001
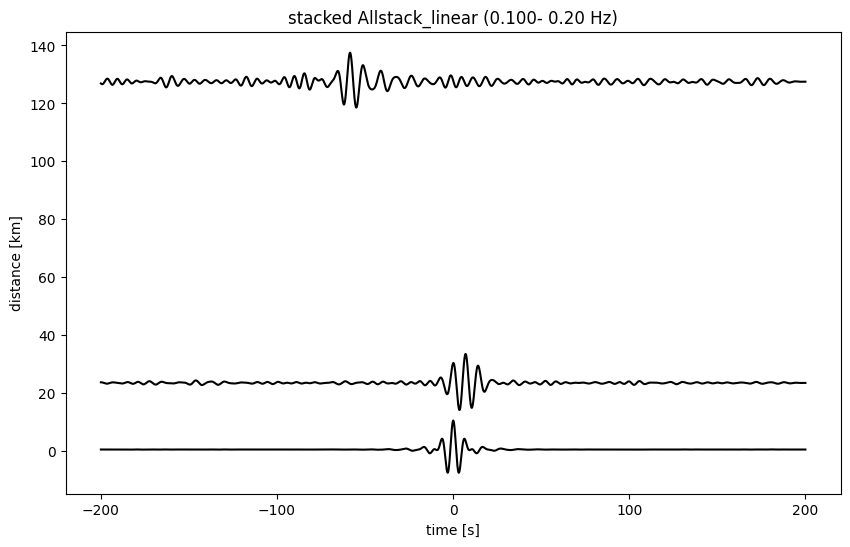